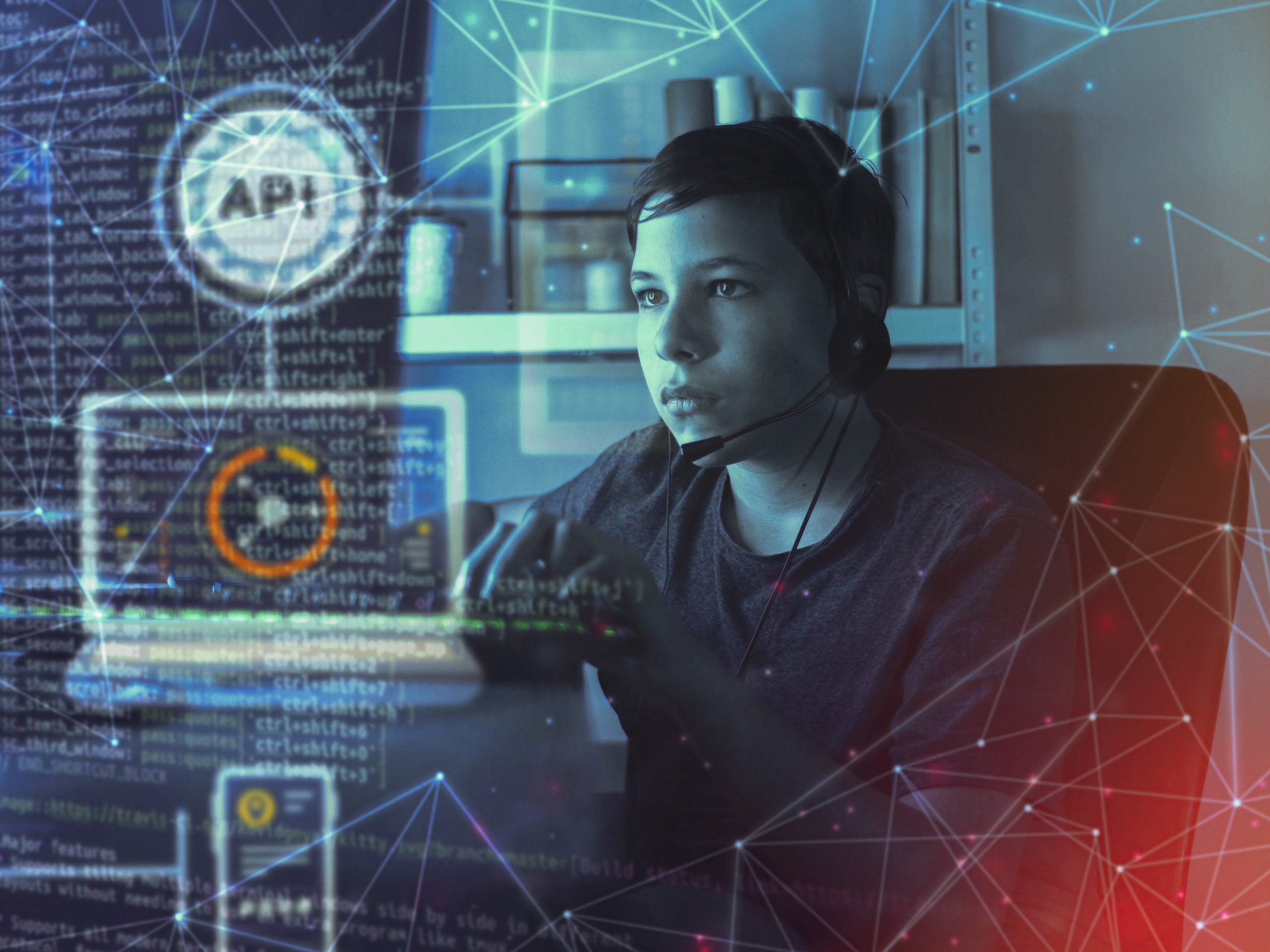
There is an increasing need to comprehend and have faith in the judgments made by intelligent systems as AI keeps seeping into more and more parts of our life. To provide light on how black box algorithms function, a new paradigm called explainable AI (XAI) has emerged. The advent of explainable AI, which provides accountability and transparency in algorithmic decision-making, is a significant step forward in a world where complicated AI models often function as incomprehensible creatures.
The Mysterious Black Box:
Many people view deep neural networks and other traditional machine learning algorithms with suspicion. It is difficult to understand how they arrive at certain judgments since their predictions are based on complex patterns and correlations within enormous databases.Concerns about biassed results, ethical issues, and AI systems' responsibility arise from this lack of transparency.
Trust through Transparency:
The goal of explainable AI is to make AI models' decision-making processes more transparent, thereby reducing the opaqueness of black box algorithms. User, stakeholder, and public confidence in XAI is enhanced by providing explanations of the reasoning behind models' conclusions and recommendations. Critical applications such as healthcare, economics, and criminal justice need this level of openness more than any other..
Understanding Models:
The creation of interpretable models is one strategy for reaching explainability. Interpretable models are built to provide clear and intelligible results, in contrast to their complicated equivalents. The intrinsic interpretability of techniques like decision trees, linear models, and rule-based systems makes them ideal for situations where transparency is of the utmost importance.
Post-Hoc Analysis:
An analysis of a black box model's output after its decision-making process is known as a post hoc explanation. Methods that draw attention to the input characteristics that affected the model's output include attention mechanisms, saliency maps, and feature importance. Post hoc explanations provide light on the decision-making process without changing the model's intrinsic complexity.
Moral Issues to Think About:
Ethical concerns about AI's possible social influence, accountability, and justice are entangled with the development of explainable AI. It is becoming more important to deploy intelligent systems in many areas while guaranteeing that their conclusions are accurate, impartial, and justifiable.
Obstacles and Ways Forward:
The creation of AI that can be explained has made progress, yet there are still obstacles to overcome. The continuing issues include, but are not limited to, finding an appropriate balance between model complexity and interpretability, solving the interpretability-accuracy trade-off, and establishing assessment measures for explainability. Improving and expanding upon current approaches is crucial for the future of explainable AI. Researchers in the field, together with ethicists and lawmakers, must work together to tackle this complex issue.
In summary:
In summary:
Interested Articles:
Why is genetic engineering considered controversial?